In January 2020, as doctors struggled to treat the first coronavirus patients, data scientists and biochemists at BenevolentAI turned to artificial intelligence to hunt for a drug to help.
The researchers had only a few clues about how the new virus operated. They fed them to their algorithm and set it to work searching 50mn medical journal articles in just a few hours. Together, the scientists and the algorithm deepened their understanding of which biological pathways a drug should target and searched for approved drugs to repurpose.
In just four days, they narrowed it down to baricitinib. The Eli Lilly drug usually used to treat rheumatoid arthritis tackles both the virus and the body’s inflammatory reaction. It was the first time AI had discovered a drug to repurpose that has then been used widely.
Peter Richardson, the vice-president of pharmacology at the London-based start-up, says AI’s prediction of how the drug would work against Covid — from its biological mechanism to antiviral effect — has now been validated. The World Health Organization strongly recommends the use of baricitinib for severe Covid and the UK’s recovery trial — the national study of treatments for the virus — found it significantly reduces deaths.
“All of that in two years,” says Richardson. “It is astonishing.”
It takes on average a decade to go from the initial discovery stage of a drug to approval and, with a high failure rate, the pharmaceutical industry estimates it costs $2.7bn to bring each one to market. Clinical trials make up the bulk of this time and cost, but discovery and pre-clinical tests can still take three or four years.

During the pandemic, AI was primarily used as a tool to save scientists’ time, accelerating this notoriously slow discovery process, while often using drugs and vaccines designed for similar viruses such as Mers as a starting point.
But advocates of AI believe its wider use during the crisis is just the start of a revolution in drug discovery that will harness growth in biological and chemical data, computing power and smarter algorithms that could reduce soaring healthcare bills and create treatments for conditions where we have none.
They argue AI proved itself in the crisis. AbCellera sorted through 6mn cells in three days to find an antibody that could be mass produced — in this case by Eli Lilly — as a drug called bamlanivimab that has helped more than 1mn Covid patients. A supercomputer helped in Pfizer’s quest for an antiviral that could be taken orally.
And although AI did not design the Covid vaccines, it optimised them. Moderna’s platform — the technology it uses for all of its vaccines — learnt from producing 20,000 unique mRNA sequences, which helped it design and manufacture the first batch of its Covid vaccine for testing in 42 days.
Venture capitalists are betting this is just the beginning. Funding for AI in drug discovery has risen 3,800 per cent in the past five years to $2bn, according to PitchBook data, and venture capital-backed start-ups have signed dozens of partnerships with Big Pharma.
Nathan Benaich, general partner at Air Street Capital, which invests in AI companies in the life sciences field, says the pandemic has reinforced the idea that drugmakers need to speed up the discovery process. “The clear benefit coming out of all this is that AI companies in this field are now pushing at much more of an open door in pharma versus before because of the obvious need for rapid innovation,” he says.
But even Benaich thinks it has not lived up to the inflated hopes at the start of the pandemic — and he is far from alone.
“I think repurposing [of drugs] is still worth pursuing,” says Eric Topol, author of Deep Medicine, a book on artificial intelligence in healthcare. “But we don’t have a lot to show for it. In the pandemic, we have one drug for sure . . . but the whole idea of AI was to take drugs and go into discovery at high velocities like we’ve never seen before.”
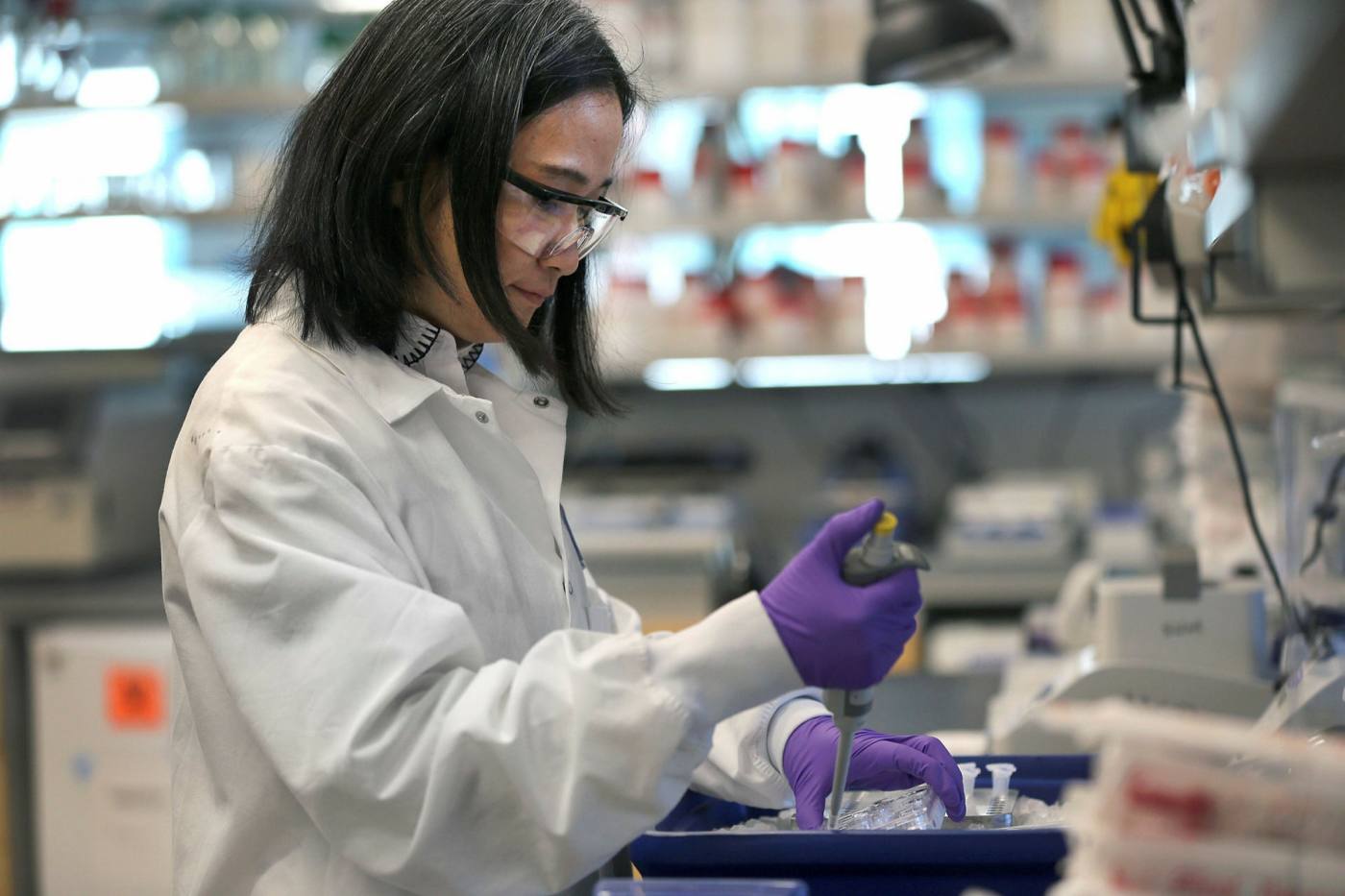
Until a regulator approves a drug discovered entirely by AI, there will be doubters. There are now 15 drug candidates designed by AI in clinical trials — but precisely because drug development can take more than a decade, it will be a long wait for a verdict.
“There’s still an awful lot of people”, says Richardson, “that don’t believe a word of it and think it’s all hype.”
Safety valves
As the pandemic progressed, clinicians around the world looked to recommendations from the UK’s recovery trial to guide their prescriptions. Martin Landray, a professor of medicine and epidemiology at Oxford university, helped run the randomised controlled trial for Covid drugs. But when selecting what to test in patients, he says, he found AI only “moderately useful”.
“The trouble is [the drug discovery algorithms] all come up with a prioritised list [of treatments] in a different order than you put in,” he says, adding the “mathematical rationale” often fails to consider practicalities.
The recovery trial’s investigators chose drugs based on having some evidence that they might work — such as tackling previous coronaviruses or proving effective in pre-clinical tests. But they also took into account whether the drug was in plentiful supply, and how much was understood about side effects.
Landray says safety has almost “infinite dimensions” that AI struggles to predict, far more complex than some of its biggest successes, such as learning to beat humans at the ancient Chinese game Go. “Instead of trying to play Go, [AI in drug discovery] is like trying to play every game that has ever been invented, some of which don’t even have rules,” he says.
Let loose on the world of biology, AI could also be dangerous. Researchers recently showed how their algorithm discovered 40,000 toxic chemical compounds, many of which could be used as biological weapons, in less than six hours.
Covid-19 showed the need for rapid drug development — but the initial lack of information about the disease meant it was not the perfect proof point for AI. At the start, there was little data for an algorithm to learn from, meaning even Benevolent’s scientists had to take a more hands-on approach — using AI as an interactive tool — than they would with other diseases.

Daphne Koller, chief executive of Silicon Valley based AI start-up Insitro, which focuses on drug discovery, applauds Benevolent for demonstrating that it is feasible to find a drug to repurpose, but warns that it is different from discovering a completely new compound.
“I’m not a fan of going out with extravagant promises about how we’re going to transform everything within three years,” she says. “Human biology is hard: intervening in something that can cause someone to die is a very risky proposition.”
Another similarly named AI start-up Insilico designed its own Covid drug. The Hong Kong-based company published a paper on its findings in April 2020 for anyone to use. When no one decided to develop it, the company synthesised the drug itself in September of the same year. But without the facilities of a large pharmaceutical company, it had to wait nine months to test it in a lab secure enough to handle the virus. And it is still yet to reach human trials.
The pandemic highlighted the two biggest hurdles for the future of AI in drug discovery: making sense of the patchy but ever more plentiful data, and changing the strategies of large pharmaceutical companies cautious about getting into bed with AI.
Frank Nestlé, Sanofi’s chief scientific officer, is embracing AI with vigour, having recently signed two big deals with Oxford-based Exscientia and medical AI company Owkin. He says AI relies on three pillars — computing power, algorithms and data.
“Data is where there’s the biggest need for improvement — and that’s also where the future step change will come,” he adds.
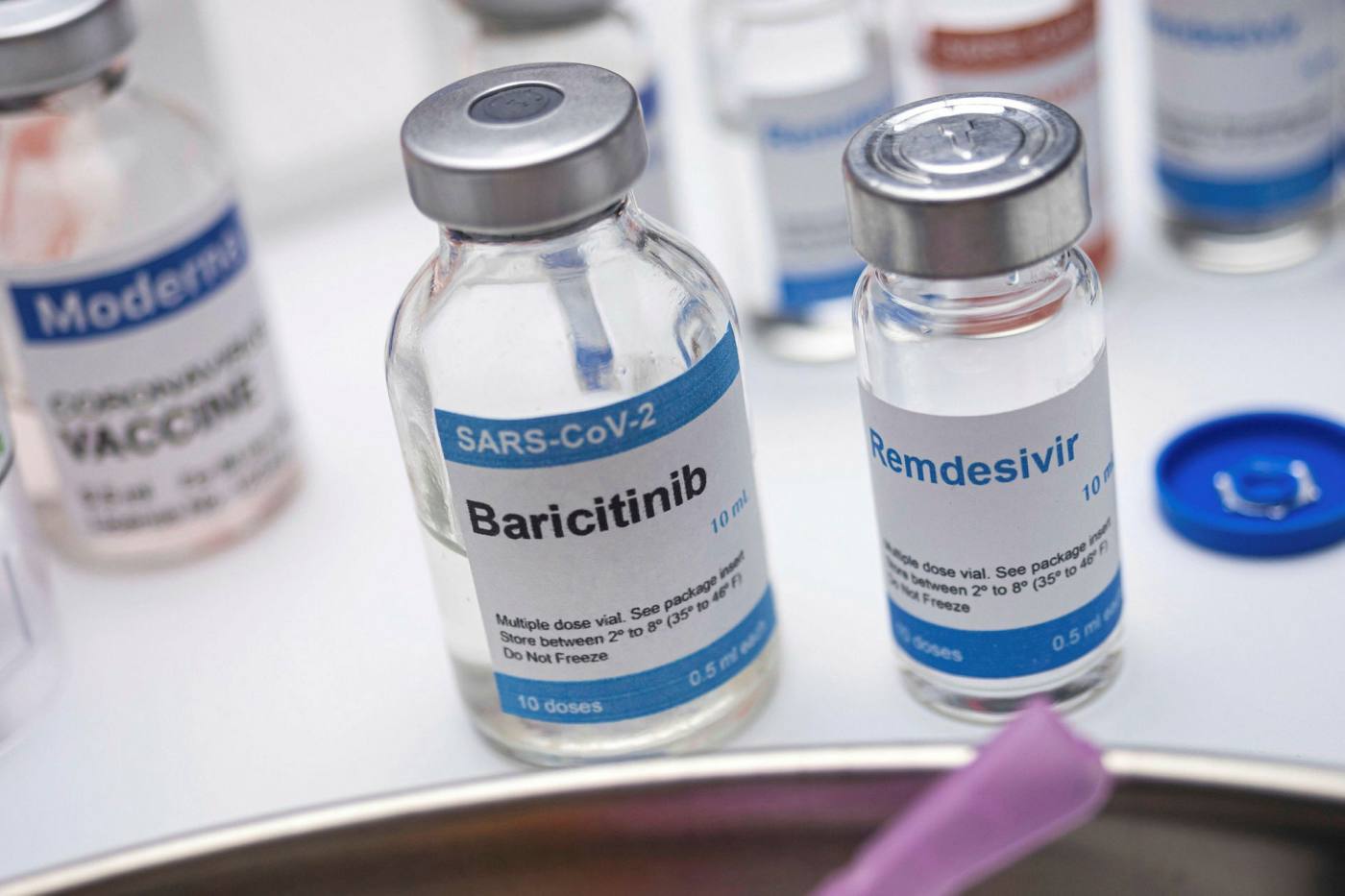
There has been an explosion of data because it has become much easier and cheaper to collect sequences of genes and proteins. Drugmakers hold huge stores of data from trials on humans and animals, but it is often in forms AI finds difficult to digest, from handwritten lab notes to Excel spreadsheets.
Nestlé says tagging this data requires painstaking precision: for example, lab samples shipped overnight should be differentiated from others, because they have different molecular footprints that could drive algorithms to the wrong conclusions based on how it was shipped, not what was in the patient’s tissue.
Such work takes time. Ugur Sahin, BioNTech chief executive, says the German biotech used a “man-in-the-middle” approach to machine learning for four years with humans helping to educate its algorithm. “AI is like kids. You have to really teach them a lot until they start to come to conclusions,” he says.
Companies that invested in their data have seen it pay dividends. In 2020, Pfizer could not automatically screen one of its libraries that holds data on 4.5bn commercially available compounds. Now, it can scan the entire database in 48 hours vastly speeding up its ability to identify potential new medicines.
Others stress the importance of hiring employees who can bridge the gap between AI and more traditional scientific methods. Najat Khan, chief data science officer and head of R&D strategy at Janssen, the pharmaceuticals arm of Johnson & Johnson, says it is building a bank of “bilingual talent”.
“We have data scientists who are highly proficient in machine learning, in AI, in digital health and real world evidence — and deeply knowledgeable in drug discovery science and medicine,” she adds.
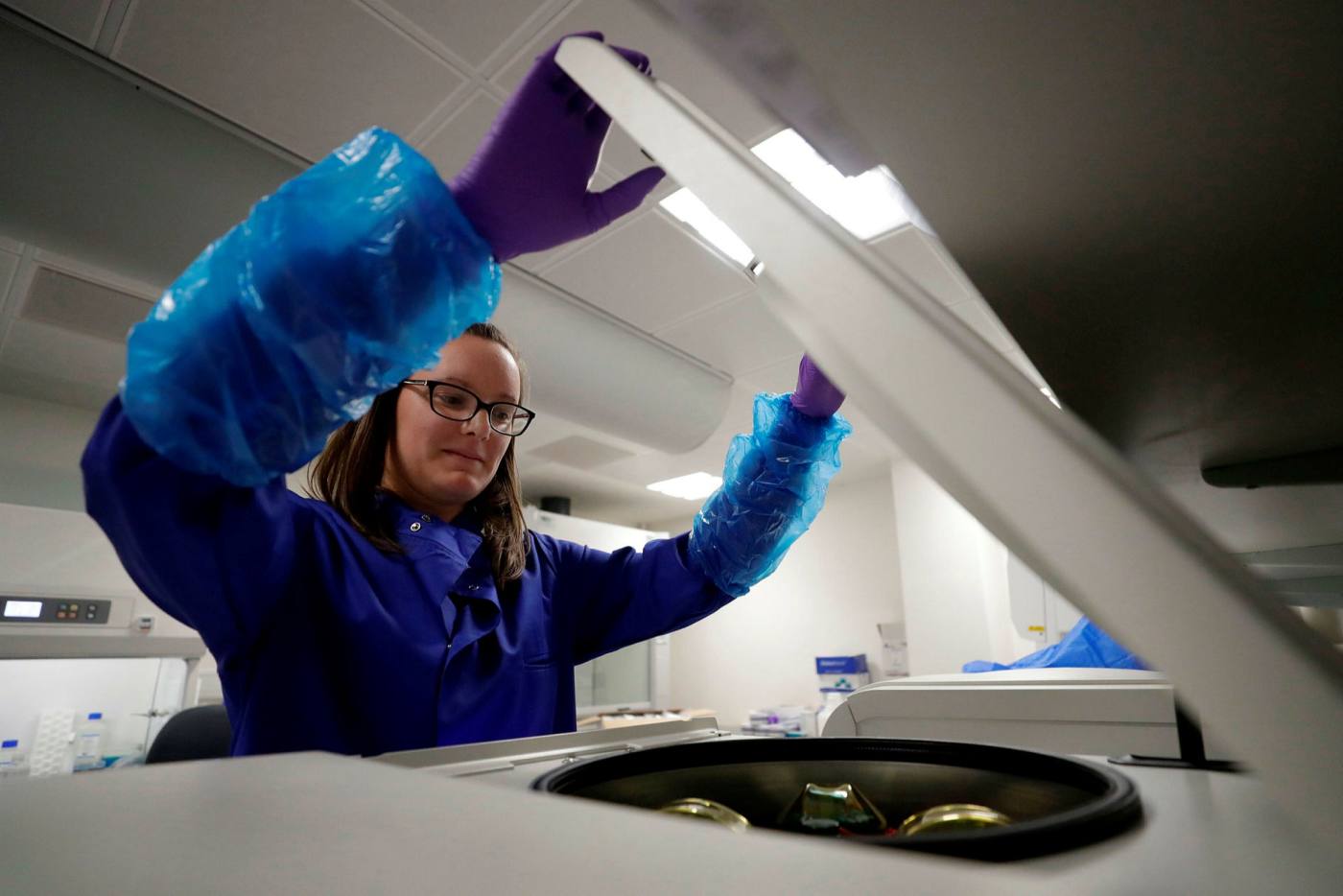
But some pharmaceutical companies are still figuring out how best to incorporate AI, unsure about how much to invest in the new field rather than progressing their current pipelines and struggling to hire talent from technology companies.
Miraz Rahman, a professor of medicinal chemistry at King’s College London, says the industry has been burnt before by pouring money into combinatorial chemistry, a method of building libraries of compounds that has been useful in recent decades but not as transformative as promised.
Yet he believes that in the next five to 10 years, all big pharma companies will have integrated AI into drug discovery. “All the data suggests AI is here to stay,” adds Rahman. “It is going to make discovery more streamlined and shave off three to four years of time if you can successfully adopt AI.”
Unlocking science
Thomas Clozel, chief executive of Owkin, says repurposing drugs is simply a “hack” and that the long-term hope for AI is that it can transform our understanding of disease.
“AI today isn’t ready to treat patients better. It is ready to accelerate the return on investment of drug discovery,” he says. “But what about augmenting it — when are you going to find things where pharma failed?”
He thinks the real innovation will come from empirical evidence mined from “patient data”. Owkin builds data sets from hospitals and analyses cellular level information to find new patient subgroups. Using computer vision — AI that can understand and read images — to study biopsies, the company can predict survival rates in patients with the aggressive cancer mesothelioma, which mainly affects the lining of the lungs. This could unlock doors for drugmakers to compounds, which might increase survival rates.
The deeper our understanding, the more personalised medicine may become. Before it became famous for its Covid vaccine, BioNTech was focused on creating “cancer vaccines” tailored to direct an individuals’ immune system to attack his or her own disease, using data from an extracted tumour. It is now ploughing ahead with that work and with AI at its core.
“The question is: if every patient has different mutations, could we build machine learning programmes that allow us to pick the best targets in each patient?” asks Sahin.
It is an “extremely complex task”, he adds, that involves weighing factors including the likelihood a mutation is in the tumour and the probability that the immune system’s T-cells recognise it. But he adds that machine learning has become “better and better”, and now humans only intervene for quality assurance.

Other innovations may accelerate progress. Last year, Google’s DeepMind released an AI tool called Alphafold2 that can predict the shape of all human proteins. By solving this huge puzzle in biology, it helps scientists understand what each protein does and how to alter it. Drugmakers can use it to understand where a drug might need to bind to a target in a human body. DeepMind plans to do just that, launching a new company Isomorphic Labs to “find cures for humanity’s most devastating diseases”.
Large pharmaceutical companies are interested in using the tool. J&J’s Khan says there are so many proteins that are considered “undruggable” because of a tricky protein. “It is going to unlock a lot of science that was impossible and make it possible,” she says.
Advances in quantum computing could also massively increase the available computer power to help tackle problems in drug discovery. But for now, AI may prove more helpful with the next generation of Covid drugs and vaccines.
The virus is now one of the most scrutinised ever. BioNTech has partnered with AI start-up InstaDeep to predict the next high-risk variant, so its flexible mRNA vaccine platform can get ahead of it. The resultant algorithm identified more than 90 per cent of variants of concern, including the Omicron strain, in advance. AbCellera used AI to select the most robust antibody that can stand up better to variants and Exscientia wants to create a broad spectrum antiviral that could be used to tackle the next pandemic virus, whatever it turns out to be.
Andrew Hopkins, Exscientia chief executive, says he is starting to see a “sea change” as larger pharmaceutical companies are more interested in investing in AI.
“There is a realisation of how we can use data and algorithms,” he says. “That what the industry did for coronavirus patients is actually something we should apply for all patients. It’s an urgency that we should bring to all medicines for all patients.”
Additional reporting by Madhumita Murgia in London